Extracting Value from Scientific Data
In recent times there has been a growing interest in analyzing data systematically to support research in scientific disciplines, using advanced techniques from data analysis and machine learning. In fact, the way in which science is carried on has evolved continuously from a pure science based on first principles to a science driven by data. The potential value of this research is very high given the quantity of data available and the possibility of generating data with sophisticated modeling tools.
Even if the approach is general since it is based on the information and process modeling that is reusable with a limited amount of adaptation across disciplines, the project focuses on the specific domain of developing simulation models for combustion kinetics relevant, for example, to the Industry 4.0 for the development of green fuels. A data-driven predictive model for combustion kinetics can study the behavior of chemicals involved in a fuel mixture and their footprint saving precious resources in terms of time and costs. Even a tiny improvement in the model’s prediction accuracy can result in better fuel efficiency and pollutants reduction.
Usually, this type of research is performed in multidisciplinary teams composed of scientists from the specific application domain and computer scientists. In our case, the main actors of this project are the Information Systems group led by prof. Pernici and the CRECK modeling group led by prof. Faravelli, both from Politecnico di Milano.
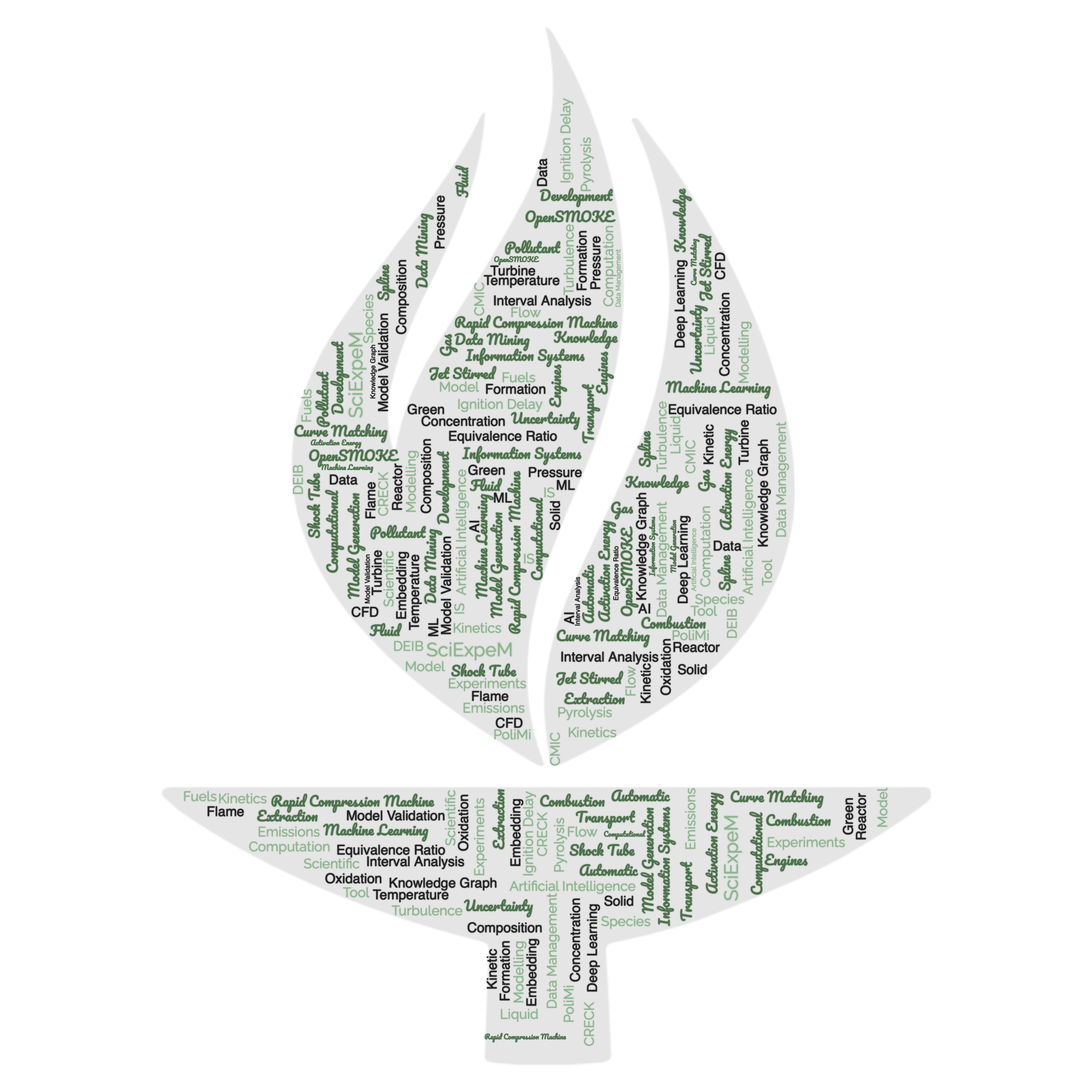
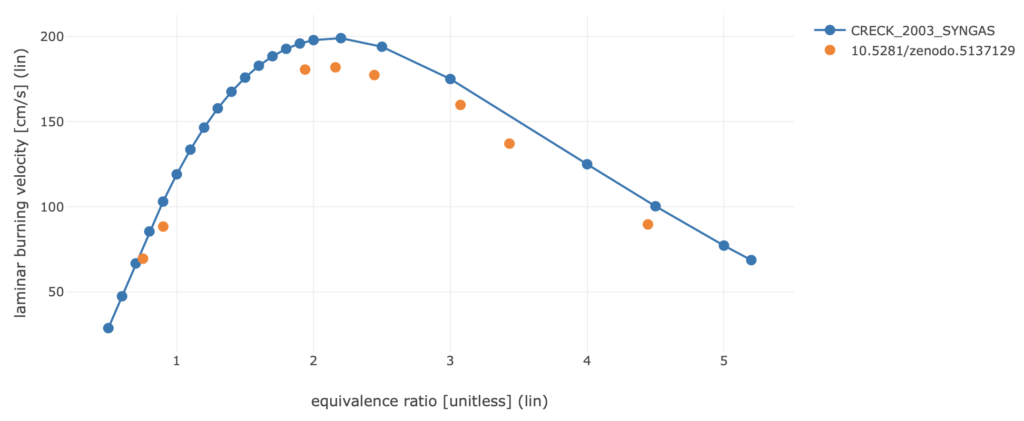
The development of predictive kinetic models requires a considerable amount of data for several aspects of the process. As in all data-driven applications, information management is a crucial aspect that often determines the quantity and quality of discoveries. This concept becomes even more true for combustion kinetics. In this domain, we have to optimize the use of simulations and experimental data, thus automating different aspects of the model development process, ranging from data collection, generation, analysis, and validation of the model itself. Many of these aspects are bottlenecks for the development process as they are very time-consuming aspects that cannot be exhaustively covered manually due to the complexity and magnitude of the problem itself. For these reasons, SciExpeM (Scientific Experiments and Models) was developed. In practice, it deals with managing pairs of simulated and experimental data trends and extracting from these, working on large numbers of data, systematicity, and valuable information for all the stages of development of the predictive model.
The first data on combustion kinetics that has been collected is dated immediately after WWII, and experimental data collection activities are still ongoing today. As one can imagine, the technologies and methods of collecting experimental data have changed over time. This change is still in progress, as neither the kinetic models nor the experimental data have reached a univocal representation format. Furthermore, scientific data are affected by a non-negligible uncertainty and other low data quality dimensions such as completeness and consistency. All these unique characteristics make this domain, but in general, any experimental domain, not easy to manage from a data point of view. For this purpose, we define a pipeline to manage scientific data in order to ensure a certain scientific repository quality. This process is merged with the model development process…
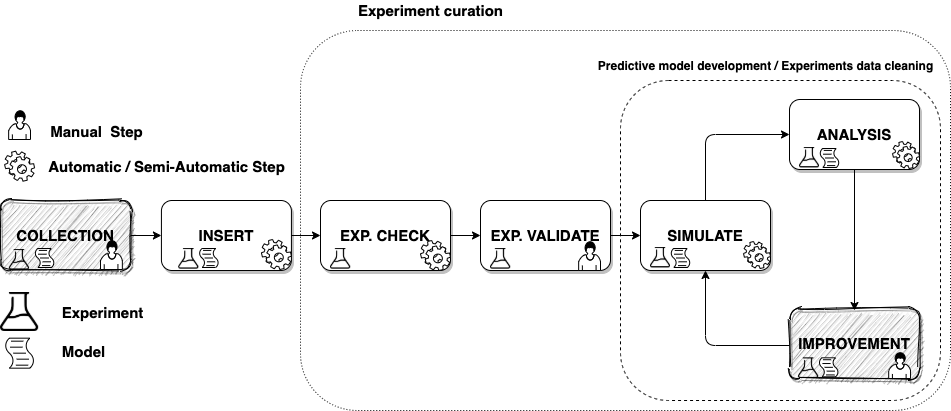
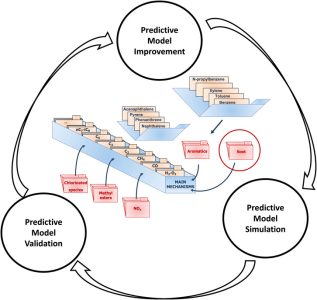